There’s no one path to creating a data strategy. Here’s how to find yours and create strong data advantages.
As the co-founder of two data start-ups, one question I get all the time is, “How do I get started with my data strategy? Where do we start? What do we prioritize?”
It’s an understandable question. Today companies are bombarded with reminders about the importance of using data. In NewVantage Partners’ annual survey, the percentage of companies that invest in data initiatives was near-universal (literally 99% in 2021) for the third year in a row.
But while investing in data is a given, actually using data can feel like a crapshoot. In that same survey, only 24% of companies said that they had created a data-driven culture.
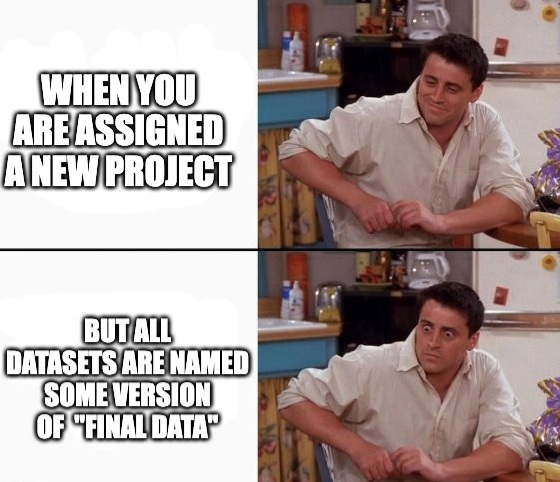
Figuring out your data strategy is far from easy. To help the leaders and companies trying to figure out their overall data strategy, I’ve created a framework that I call the Data Advantage Matrix.
In this article, I’ll break down how to think about your data strategy, how the matrix works, and give examples of how two hypothetical companies would use it.
Data strategy isn’t linear or universal
There are plenty of resources that promise the one true path to creating a data strategy. “Do these 5 things and you’ll become a data-driven company” or “These are the 5 steps to monetizing your data” and so on.

I wish it was that simple.
Here’s the painful truth that I’ve learned from running countless data projects: There’s no one path to creating a data strategy. Every company is unique, every business is unique, every industry is unique, and so every company’s path is going to be unique. Rather than looking at what other companies have done, the key is examining your own needs and prioritizing the best data investments for your company
How can you actually know what you should prioritize? Many resources talk about focusing on projects with the highest ROI (i.e. the return that these initiatives will bring your company).
However, I believe that this is the wrong way to look at your data strategy. Because let’s face it, every data person knows that your inputs and assumptions drive the final output of your ROI calculation. I believe that companies shouldn’t prioritize data projects from an ROI lens, but instead an “advantage” lens.
Which data investments will help you build sustainable competitive advantages to outperform your competitors?
The Data Advantage Matrix
The Data Advantage Matrix can help you figure out what types of data advantages you want to build and how far you want to advance them.
Here’s how it works — the rows represent the type of data advantages that companies can create, and the columns are the three stages for each of those advantages.
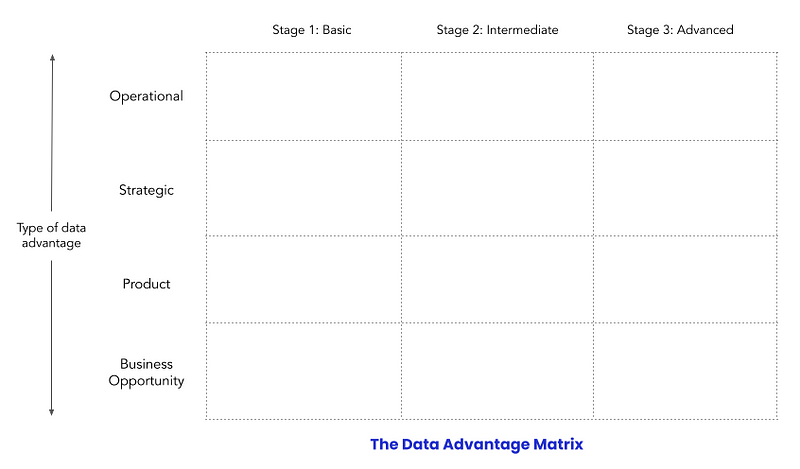
Let’s break this down, starting with the four types of data advantages:
- Operational: This is about understanding the levers that drive your business, then using them to improve operations. A key aspect is making data available and understandable to those who are making daily decisions. An example is Gojek’s daily updates about key metrics, which its first CEO Nadiem Makarim used to form an intuitive sense of what was breaking.
- Strategic: Every company makes a few critical strategic decisions each year. The more data-driven these decisions are, the more likely that they will jumpstart growth or success. An example is the Government of India using geo-clustering to open 10,000 new LPG centers.
- Product: This is when companies leverage data to drive a core product advantage, one that separates them from competitors. An example is Google’s “smart compose” auto-completion feature.
- Business opportunity: This involves using company data to find and create new business opportunities. An example is Netflix Originals, where Netflix started to produce its own TV shows and movies based on its data about what people want to watch.
I’ve already written about these four types of advantages, so check out that article if you want to learn more.
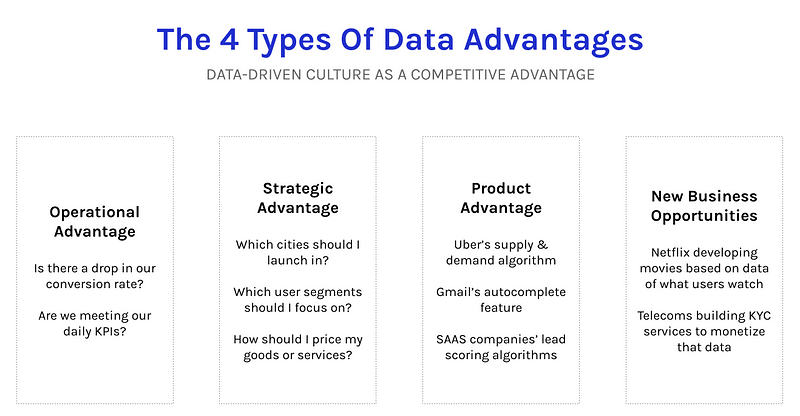
Next, we’ve got the three stages of each data advantage:
- Stage 1 (Basic): This is a quick-and-dirty MVP that uses basic tools (e.g. SAAS products, Google Sheets, Zapier) and no data specialists. I almost always recommend that companies start new initiatives in the “Basic” tier to quickly deploy and assess a solution.
- Stage 2 (Intermediate): This tier includes investments in data platform tooling and data specialists or teams.
- Stage 3 (Advanced): These are best-in-class data initiatives with specialized teams for each use case or project. When you get to this tier, you’re basically a case study for what it means to be a data-driven company.
Ready to see this in action? Let’s walk through a couple of examples to see how two hypothetical companies would use the Data Advantage Matrix to set their data strategy.
Example #1: Using the matrix to prioritize data initiatives for a SAAS software startup
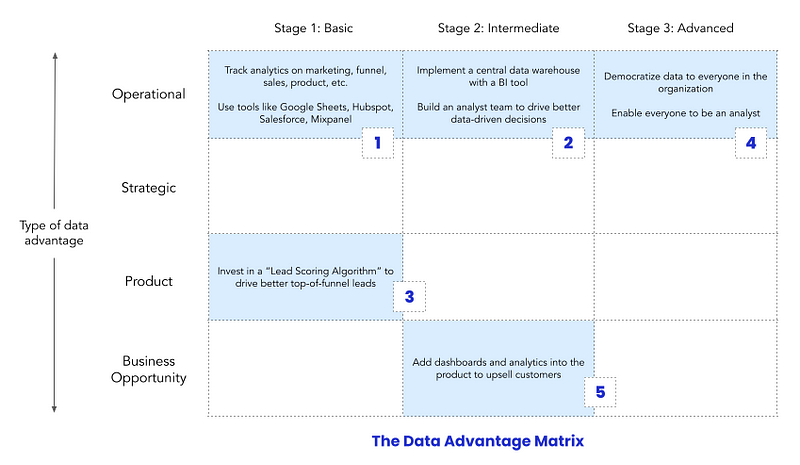
For SAAS companies, the funnel is everything. Optimizing metrics at every stage of the funnel is what accelerates SAAS companies from average to exponential growth. So for any SAAS founder, if you don’t have basic operational analytics set up on day one, you’re probably doing something wrong.
This fictional SAAS startup would start at the top left of the matrix with basic operational analytics. These analytics don’t have to be complicated. At Stage 1, it’s all about getting the basics right — measuring the number of leads per day, users converting on the site, users signing up on the product, free trials that end up paying, etc.
Given the importance of operational analytics, it would make sense for this startup to move to Stage 2 pretty quickly — converting its basic analytics into something more scalable like a centralized intelligence engine. This would include investing in a data warehouse (like Redshift or Snowflake) that brings all data into one place, adding a BI tool, and hiring the first analysts to drive data-driven decisions where it matters most (like marketing or SalesOps).
At this point, with operations sorted, the startup can start thinking about other aspects of the business:
- To drive outbound sales, it can invest in building a moat against competitors, like a lead-scoring algorithm to help sales reps focus on the best leads.
- It could move back to its core advantage, operational analytics, and make that super data-driven and democratized.
- It could even focus on a new advantage, like creating a dashboard inside its product to upsell customers.
Example #2: Prioritizing data initiatives for a cab aggregator
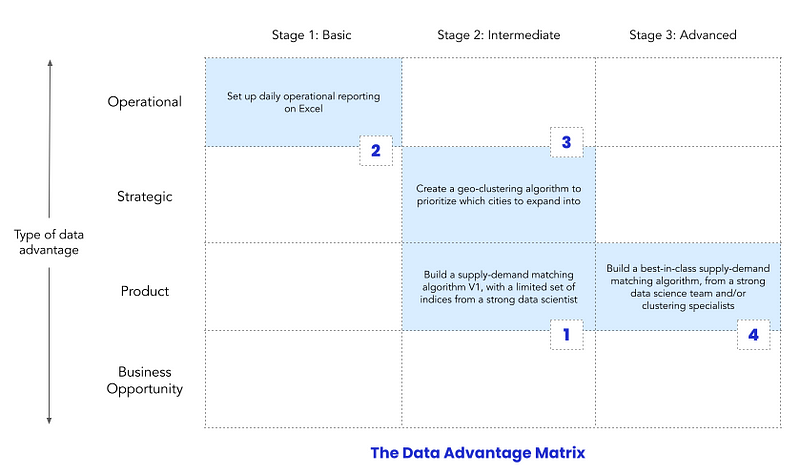
Let’s take on another example, a cab aggregation startup.
This is a completely different situation because a cab company’s entire product depends on balancing supply and demand. If customers can’t find a cab, they won’t find value in the cab company.
That’s why a cab aggregator would start in a different place from a SAAS company — a product advantage. It would first invest in building a supply-demand matching algorithm, so its third or fourth hire would need to be a strong data scientist to build proper tooling and make its algorithm a competitive advantage on day one.
Next, it might make sense for the company to build basic operational reporting on something like Excel. Then it might make sense for the company to take on strategic questions like how to expand from one city to ten cities. Since this is a critical question, it could again skip to Stage 2 — investing in a data scientist to build a proper geo-clustering algorithm.
Along the way, the cab startup would likely want to upgrade its supply-demand matching algorithm and build a strong team and best-in-class data system, making this an advanced data advantage.
Using the Data Advantage Matrix for your company
So what should the Data Advantage Matrix look like for your company?
Start by thinking about what you want your competitive advantage to be. What will enable you to out-execute your competitors?
List all of the data advantages that you can build into your business, and then think about which you want to take on. Does it make sense to focus on upgrading your core advantage, or is it better to launch “basic” data initiatives across other types of data advantages?
There is no straightforward path to prioritizing your data investments. For example, two similar SAAS startups might take different approaches to build their data strategy and team. When you think about prioritizing possible initiatives, start with three fundamental questions:
- What kind of data advantage will this create?
- How could this initiative impact our company?
- How much effort will it take for us to get there?
Note that data advantages don’t always have to start at Stage 1. Like the cab aggregator, it might make sense to start at Stage 2 if a data advantage is critical to your company and can be built on proper tooling from the start. When you can start with something quick and dirty, figure out exactly what you need, and then upgrade over time, start at Stage 1 with an MVP — which is what I usually recommend.
Looking back on hundreds of data projects
In my experience working with data leaders on over 200 data projects, I’ve learned is that there’s no one linear path to creating a data strategy for your company. The one thing that’s paramount is to start with first principles.
Remember that your business is unique, and so the data moats and advantages that you create for yourself will be unique from every other company in the world.
When you think about finding your data strategy, start at the basics. Consider the different types and levels of advantages that you could build, start with the lowest hanging fruit that can create a meaningful impact, and just keep iterating from there.
As you embark on creating these data initiatives, remember to build the right foundation. Don’t forget to invest in the data tools you need, and set up a modern data platform whenever you hit Stage 2. Data access and collaboration are optional at Stage 2, but definitely make sure you figure them out by Stage 3.
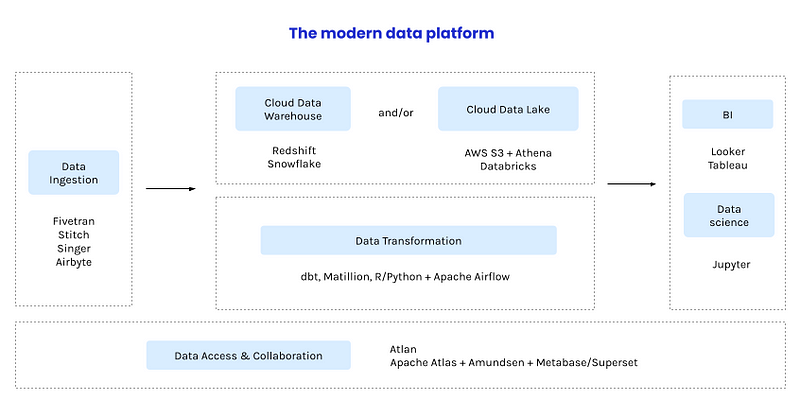
Good luck with your journey, and drop a comment if you have any questions!
This article was originally published on Towards Data Science.